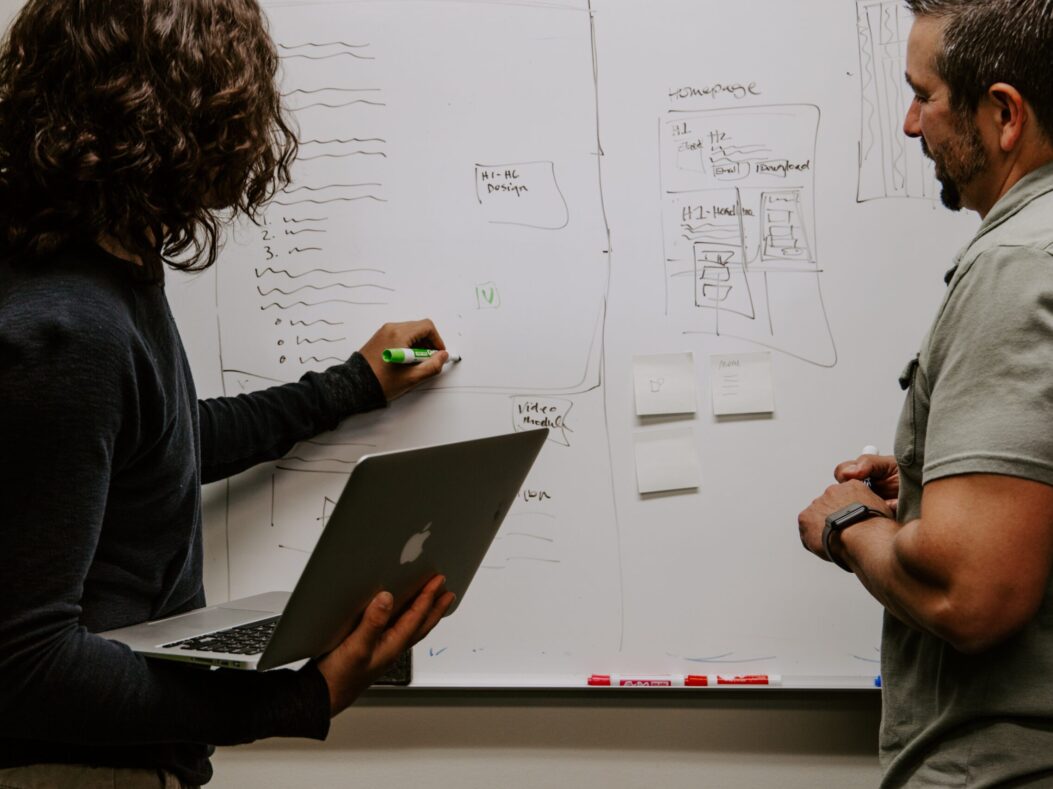
4 Essential Steps for Building Scalable Data-Driven Products
An eager data scientist exclaims to the product manager, “Imagine the possibilities!” The product manager looks at him and asks, “What does it do?” The data scientist says, “We don’t know yet, but just imagine the possibilities!”
Some may laugh at this conversation, others may cringe at the recent memories it invokes, and still others may utter involuntary frightful screams. Perhaps the relationship between product managers and data scientists is a necessary evil, a perpetually at odds system of checks and balances that attempts to ensure superior products for your customers and overall success of the business.
So what does one do with such a recipe for frustration to scale it? Is it even possible? It is! In this post I will share the 4 essential steps to building scalable data-driven products:
Teach how to learn the outcome.
Effectively communicate big data.
Give them a seat at the strategy table.
Carryout your proper roles.
1. Teach How to Learn the Outcome
Sitting around the table of a data-driven product team, you will have a cacophony of masters degrees, expertly honed skills, and big brains. So it might seem counterintuitive that a key responsibility of the product manager is to teach them how to learn. Yet, learning how to learn the outcome is perhaps the most vital point of creating a scalable data-driven product.
One effective method to teach outcomes is to not let your data scientist team look at the data set. Instead, first introduce them to a myriad of webinars, conferences, blog articles, etc. that talk about many of the problems businesses are solving with data-driven products. Reason being, many of these presenters are trained in communicating large complex issues and their equally large complex solutions to business executives through the language of the business problem. Next, allow your team members’ minds to run wild. Let them choose the business problem that interested them most and then have them go figure out how that particular company did it. There are many helpful scholarly journals and research forums with information in this area. Finally, present your customer to your team, they will listen to the problem completely detached from the type of data you have. But why should you do this incredibly reverse way of orienting your data team before showing them the data set? Simple, data sets can always be changed, but customer needs cannot.
2. Effectively Communicate Big Data
Tara Syed Williams, is the Director of Business Analytics at Pinterest.com, in her presentation on Scaling Business Analytics at Pinterest she states that the responsibility of her team is to “Translate data into what makes strategic sense for the business.” To best accomplish this she says, “We have to make big data feel small.” especially when communicating complicated information to the CEO. Taking notes from the presentations in Step 1 will enable your data team to see how very complicated methods are communicated in simple and digestible formats. Joseph Kong is the Senior Data Scientist at Trulia.com, and he gives a fantastic presentation (Better Consumer Experiences with Applied Machine Learning) that communicates complicated machine learning in a format that is clear and digestible to broad range of individuals.
3. Give Them a Seat at the Strategy Table
It is one challenge to identify the business problem, it is another to identify the right business problem. Companies change their strategies many times over, refocusing a team on a different consumers many times over. Above and beyond communication from the strategy team is vital to allowing data teams to refocus properly. Discovering a solution takes time, the more time the strategy team can dedicate to the data team, the more success is possible with a scalable data-driven product.
Tara Syed Williams also talks about how her team stays informed of the strategy changes at Pinterest. Each week the executives meet with the purpose of ensuring everyone knows what is going on. They identify opportunities, manage metrics, and give updates on projects. Also, every other week, the teams each do a presentation to see how they are performing against their quarterly roadmap and the executives give input and adjust the direction of the projects as needed. Corporate strategic insight is vital for a team’s success to both know what to work towards and to see how they can positively affect the overall direction of the company.
4. Carryout Your Proper Roles
Vikas Sabnani is the Senior Director of Data Science and Analytics at Glassdoor.com. In his presentation Building Data Products-There’s More it than Just Data, he traces the history of the products at Glassdoor.com and how each function on the data team played a role. They setup the usual structure, from setting benchmarks for the data-scientists to achieve, to the product managers focusing on understanding the customer etc. Yet, the key insight from his presentation is what happened when a bug hit their product that actually benefitted the business. The product manager was overjoyed, the data scientist was fascinated and wanted to know why it happened, and the engineer was mortified and wanted to fix the bug. Many of their exploits in successfully launching Glassdoor off the ground are around the same issue, who occupies what role on the data team. Sometimes it was confusing, other times it was simply being agile, but Glassdoor persevered solving the relationship between the data scientist and product management and a strange thing happened…innovation.
Scalable does not come from product managers, it comes from data scientists. Innovative does not come from data scientists, it comes from product managers. It is important for the data scientists to understand the customer and the business strategy, yet their chief role is to determine how to fulfill both with a data-driven product that works well for any number of users. It is equally important for the product manager to enable the data scientist in this capacity and direct the product in more than just a way that satisfies the customer, but also in a way that is innovative, new, and superior to all other scalable data-driven products.